Conducting and Presenting SPSS Data Analysis for Research Projects
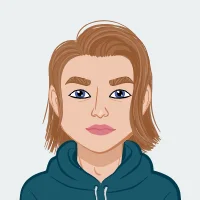
Successfully completing assignments involving SPSS output and statistical analysis requires a clear understanding of the processes involved. To solve your SPSS assignment effectively, you need to navigate through various steps, from comprehending the research question to presenting your results professionally. This guide will walk you through the essential aspects of SPSS data analysis, including selecting appropriate statistical methods, generating fictitious data, conducting a priori and post hoc analyses, and using SPSS for accurate data interpretation. By following a systematic approach, you can ensure that your work is thorough, precise, and professionally presented, making your research valuable and impactful. Whether you are a beginner or looking to refine your skills, this comprehensive guide will provide the insights needed to do your statistics assignment with confidence and accuracy.
1. Understanding the Research Question
The first and most crucial step in solving your SPSS assignment is to thoroughly understand the research question. This involves breaking down the question to identify its core components and the relationships between them. Here’s how you can effectively comprehend and approach the research question:
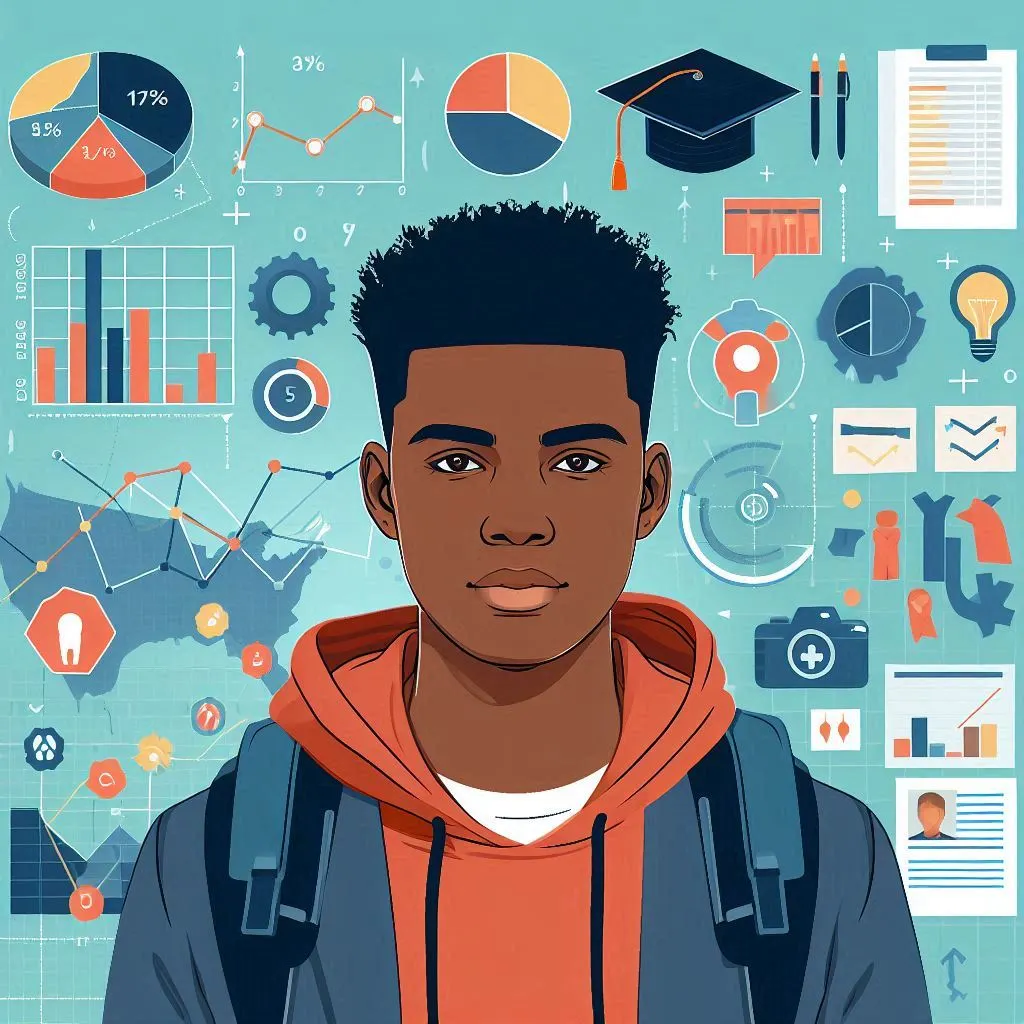
Identify the Purpose
What is the main issue or phenomenon being investigated? Understanding the broader context of the research helps you align your analysis with the intended outcomes.
Determine the Key Variables
Next, pinpoint the key variables involved in the research. These typically include:
- Independent Variable (IV): This is the variable that you manipulate or categorize to observe its effect on the dependent variable. For example, different types of recovery training classes.
- Dependent Variable (DV): This is the outcome variable that you measure. It is influenced by changes in the independent variable. For instance, the number of relapses in a substance abuse recovery program.
- Covariates: These are additional variables that might influence the dependent variable and need to be controlled for. An example could be the participants' biblical knowledge.
Formulate Hypotheses
Hypotheses are predictions about the relationships between variables. They can be directional (predicting a specific outcome) or non-directional (predicting a general relationship). For example:
- Null Hypothesis (H0): There is no significant difference in the number of relapses among the different types of recovery training classes.
- Alternative Hypothesis (H1): There is a significant difference in the number of relapses among the different types of recovery training classes.
Understand the Research Design
Determine the research design that will best address the research question. This includes understanding the type of study (e.g., experimental, correlational), the sampling method, and how the variables will be measured and analyzed. In many SPSS assignments, you may be provided with a specific design to follow, such as ANCOVA, ANOVA, or regression analysis.
Consider the Data Requirements
Think about the data needed to answer the research question effectively. This includes the sample size, the method of data collection, and the type of data (e.g., continuous, categorical). Ensure that the data you collect or generate aligns with the requirements of your chosen statistical test.
2. Selecting Appropriate Statistical Analyses
Choosing the right statistical analysis is a critical step in solving your SPSS assignment. The choice depends on the nature of your research question, the types of variables involved, and the hypotheses you aim to test. Here’s a detailed guide to help you select the most appropriate statistical analyses for your research:
Understand Your Variables
Before selecting a statistical test, it’s essential to understand the types of variables you are working with:
- Independent Variables (IV): These are the variables you manipulate or categorize to observe their effect on the dependent variable. They can be categorical (e.g., type of recovery training class) or continuous (e.g., dosage levels).
- Dependent Variables (DV): These are the outcome variables you measure, which are affected by the independent variables. They can also be categorical (e.g., pass/fail) or continuous (e.g., test scores).
- Covariates: These are additional variables that might influence the dependent variable and need to be controlled for in the analysis (e.g., age, gender, pre-existing knowledge).
Match Analysis to Research Question
The nature of your research question will guide the selection of the statistical test. Consider the following types of questions:
- Comparing Groups: If your research involves comparing means between two or more groups, you might use t-tests, ANOVA, or ANCOVA.
- Relationship Between Variables: For questions exploring relationships between variables, consider correlation or regression analyses.
- Prediction: If you aim to predict outcomes based on one or more predictors, regression analysis is appropriate.
- Frequency and Proportions: For categorical data involving frequencies or proportions, chi-square tests are suitable.
Common Statistical Tests
Here are some common statistical tests and when to use them:
- t-Tests: Use t-tests when comparing the means of two groups. There are independent samples t-tests (for two separate groups) and paired samples t-tests (for two related groups).
- ANOVA (Analysis of Variance): Use ANOVA to compare the means of three or more groups. One-way ANOVA is for a single independent variable, while two-way ANOVA is for two independent variables.
- ANCOVA (Analysis of Covariance): Use ANCOVA when you want to compare the means of three or more groups while controlling for one or more covariates.
- Regression: Use regression analysis to predict the value of a dependent variable based on one (simple regression) or more (multiple regression) independent variables.
- Chi-Square Test: Use chi-square tests to examine the relationship between two categorical variables.
By carefully selecting the appropriate statistical analyses and ensuring that all assumptions are met, you can effectively solve your SPSS assignment and produce valid, reliable results. This approach not only helps in answering your research question but also enhances the credibility and accuracy of your findings.
3. Conducting A Priori and Post Hoc Analyses
In the realm of statistical analysis, both a priori and post hoc analyses play crucial roles in ensuring the robustness and reliability of your research findings. To complete your statistics assignment involving SPSS effectively, it’s essential to understand how and when to conduct these analyses. This section provides a detailed guide on executing a priori and post hoc analyses.
A Priori Analysis
A priori analyses are conducted before the data collection phase. They help in planning your study by determining the necessary sample size to achieve adequate statistical power, thus ensuring that your study can detect meaningful effects if they exist.
Steps in A Priori Analysis
- Define Your Hypotheses: Clearly outline your research hypotheses, including the expected direction of the effect (e.g., one-tailed or two-tailed test).
- Specify Effect Size: Estimate the expected effect size based on previous research or pilot studies. Effect size is a measure of the magnitude of the relationship or difference being studied.
- Determine Significance Level (α): Decide on the significance level (commonly set at 0.05), which is the probability of rejecting the null hypothesis when it is actually true.
- Select Desired Power (1-β): Choose the desired power level (commonly 0.80 or 80%), which is the probability of correctly rejecting the null hypothesis when it is false.
- Calculate Sample Size: Use statistical software or power analysis tools like G*Power to calculate the required sample size based on the specified parameters.
Using SPSS for A Priori Analysis
While SPSS does not directly provide tools for a priori power analysis, you can use external tools like G*Power to perform these calculations. Input the effect size, significance level, desired power, and number of groups or predictors to obtain the recommended sample size.
Post Hoc Analysis
Post hoc analyses are conducted after the primary analysis to explore specific group differences or relationships that were not specified before the study. These analyses help in understanding the nuances of the data and ensuring that the observed effects are not due to random chance.
Steps in Post Hoc Analysis
- Perform Primary Analysis: Conduct your primary analysis (e.g., ANOVA, ANCOVA) to identify significant main effects or interactions.
- Check Assumptions: Ensure that the assumptions of your primary analysis are met, as violating these assumptions can affect the validity of post hoc tests.
- Select Post Hoc Tests: Choose appropriate post hoc tests based on your primary analysis. Common post hoc tests include Tukey’s HSD, Bonferroni correction, and Scheffé’s test.
- Control for Type I Error: Use post hoc tests that adjust for multiple comparisons to control for the increased risk of Type I error (false positives).
- Interpret Results: Carefully interpret the results of the post hoc tests, focusing on the adjusted p-values and confidence intervals to understand the specific group differences or relationships.
By conducting thorough a priori and post hoc analyses, you can solve your SPSS assignment with greater precision and confidence. These analyses not only enhance the validity of your findings but also provide deeper insights into your research data.
4. Using SPSS for Data Analysis
SPSS (Statistical Package for the Social Sciences) is a powerful software tool used for statistical analysis. When working on your SPSS assignment, mastering the use of SPSS for data analysis is crucial. Here’s a detailed guide to help you effectively use SPSS to analyze your data and solve your SPSS assignment.
Setting Up Your Data
1. Inputting Data
- Create a New Data File: Open SPSS and create a new data file. You can do this by navigating to File > New > Data.
- Define Variables: In the Variable View tab, define your variables by entering their names, types (e.g., numeric, string), and labels. Specify measurement levels (e.g., nominal, ordinal, scale) for each variable.
- Enter Data: Switch to the Data View tab and enter your data. Each row represents a case (e.g., participant), and each column represents a variable.
2. Data Cleaning
- Check for Missing Data: Use the Analyze > Descriptive Statistics > Frequencies function to identify missing data. You can handle missing values by imputation or exclusion, depending on the analysis.
- Identify Outliers: Use graphical methods like boxplots (Graphs > Chart Builder) or statistical methods to detect and handle outliers that could skew your results.
Performing Descriptive Statistics
1. Descriptive Analysis
- Summarize Data: Use Analyze > Descriptive Statistics > Descriptives to obtain summary statistics such as mean, median, standard deviation, and range.
- Explore Data: Use Analyze > Descriptive Statistics > Explore to generate descriptive statistics and visualizations for continuous variables.
Conducting Inferential Statistics
1. Hypothesis Testing
- T-Tests: To compare the means of two groups, use Analyze > Compare Means > Independent-Samples T Test for independent groups or Paired-Samples T Test for related groups.
- ANOVA: To compare means across three or more groups, use Analyze > Compare Means > One-Way ANOVA for a single factor or Analyze > General Linear Model > Univariate for multiple factors.
- ANCOVA: To control for covariates while comparing group means, use Analyze > General Linear Model > Univariate and include covariates in the model.
2. Correlation and Regression
- Correlation Analysis: To examine the relationship between two continuous variables, use Analyze > Correlate > Bivariate and select Pearson or Spearman correlation.
- Regression Analysis: To predict a dependent variable based on one or more independent variables, use Analyze > Regression > Linear for simple or multiple regression models.
Interpreting and Presenting Results
1. Analyzing Output
- Understand SPSS Output: Carefully examine the SPSS output, focusing on key elements such as p-values, confidence intervals, effect sizes, and test statistics.
- Narrative Interpretation: Provide a clear narrative interpretation of the results, explaining what the statistical outputs mean in the context of your research question.
2. Creating APA-Formatted Tables and Figures
- Exporting Tables and Figures: SPSS allows you to export tables and figures in formats compatible with APA style. Use File > Export to save your outputs.
- Formatting: Follow APA guidelines to format tables and figures. Include descriptive titles, labels, and notes as necessary.
3. Writing the Results Section
- Structure: Organize the results section by research questions or hypotheses. Present descriptive statistics first, followed by inferential statistics.
- Clarity: Ensure that your writing is clear and concise, making it easy for readers to understand the statistical findings and their implications.
By effectively using SPSS for data analysis, you can confidently solve your SPSS assignment. This process involves setting up and cleaning your data, performing descriptive and inferential statistics, checking assumptions, and interpreting and presenting your results. Mastering these steps ensures that your research is robust, valid, and clearly communicated.
Conclusion
Solving your SPSS assignment involves more than just running analyses; it requires a thorough understanding of each step in the process. By comprehensively understanding the research question, selecting appropriate statistical tests, generating realistic fictitious data, and conducting both a priori and post hoc analyses, you can ensure that your results are valid and reliable. Presenting your findings in a professional manner, adhering to APA formatting standards, and creating clear visual aids will further enhance the impact of your work. This structured approach not only helps in solving your SPSS assignment but also equips you with the skills needed for future research projects. With practice and attention to detail, you can confidently tackle any SPSS-related task, making your research meaningful and accessible to others.